Operationalizing Large Language Models for Healthcare: Key Technical Challenges
- This article, authored by Ankit Virmani, a Forbes Technology Council member, and Agbolade Omowole, an Agenda Contributor for the World Economic Forum, discusses the use of LLMs in healthcare
- AI experts have listed the challenges facing the use of AI in healthcare as large language models become a thing
- Ankit and Agbolade noted that LLM errors in healthcare use cases could have dire consequences. As such, rigorous model testing regimes adapted from software engineering and AI safety best practices are critical
Large language models (LLMs) like ChatGPT represent a transformative AI capability with profound potential for the healthcare sector. By ingesting and contextualising massive datasets, LLMs can aid clinicians in diagnosis, treatment selection, medical research, and more. However, using these systems requires addressing significant technical hurdles spanning data quality, model interpretability, robust testing, and data privacy.
The predictive prowess of healthcare LLMs hinges on their training data, which encompasses electronic medical records, clinical literature, treatment guidelines, and more.
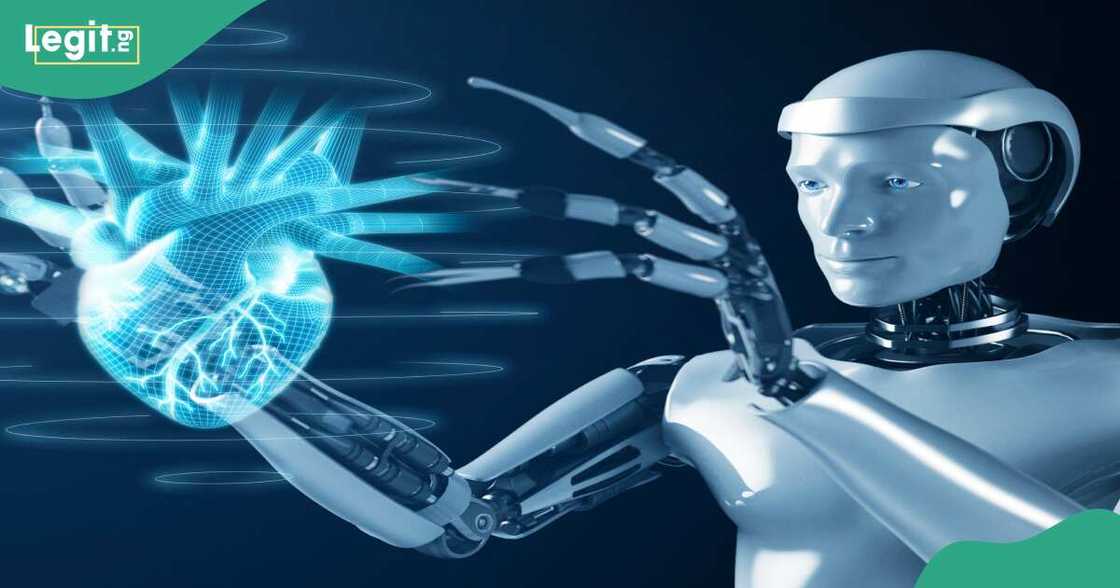
Source: Getty Images
AI biases are based on human biases
Unfortunately, many existing datasets exhibit demographic and socioeconomic biases that could be amplified if addressed during model training. According to Agbolade Omowole, the founder of the Global AI Ethics Conference, AI Bias exists because biased humans code them.
PAY ATTENTION: Сheck out news that is picked exactly for YOU ➡️ find the “Recommended for you” block on the home page and enjoy!
Data science teams must implement rigorous protocols to audit training data for representation disparities and broader bias evaluation using techniques like subgroup replication analysis.
Omowole said that biased data can lead to poor health diagnosis outcomes for under-represented people such as Africans. He suggests that LLMs working in healthcare should be trained on more data from Africans and blacks to balance their representation in the training dataset.
Despite their sophistication, LLMs are complex "black boxes" that can generate flawed yet persuasive outputs. When advising on life-impacting medical decisions, the rationales behind LLM recommendations must be interpretable to human domain experts exercising final judgment, such as doctors.
Data scientists should focus on developing interpretable LLM architectures that expose intermediate reasoning steps through attention flow visualization and other model-agnostic explainability methods. Clinicians must understand LLM confidence levels and failure modes across different contexts. Promising techniques from areas like knowledge distillation and neural-symbolic computing may bridge the "explainability gap" between LLMs and human reasoning.
Key challenges
LLM errors in healthcare use cases could have dire consequences. As such, rigorous model testing regimes adapted from software engineering and AI safety best practices are critical, including:
- Generative stress testing across broad clinical scenarios
- Probing for inconsistent outputs, nonsense reasoning, or hallucinated knowledge
- Monitoring for model drift as new data is incorporated
- "Red teaming" frameworks to uncover edge cases and vulnerabilities
Validated monitoring systems and "killswitches" must rapidly respond to detected issues. Test-driven LLM development and observability pipelines will be vital for maintaining model integrity.
Pathway to overcoming the challenges
LLM training data includes sensitive Protected Health Information (PHI). Healthcare organizations must implement robust data governance protocols, storage, and compute infrastructure to preserve privacy and prevent unauthorized PHI exposure.
According to Ankit Virmani, an experienced professional who works on Ethical AI Systems and is a member of the Forbes Technology Council, the path toward healthcare LLM adoption is multifaceted. It requires coordinated efforts spanning data quality assurance, model interpretability, rigorous testing frameworks, and stringent privacy-preserving protocols.
Ankit Virmani believes that by proactively addressing these interconnected challenges, the healthcare sector can harness AI's potential while centering on patient well-being, equity, and trust.
Young Nigerian AI expert rolls out mentorship plans for youths
Legit.ng previously reported that Artificial Intelligence and Machine Language expert Oludayo Ojerinde has unveiled a scheme to guide young individuals keen on mastering artificial intelligence.
Ojerinde, also the brain behind Davirch AI Consult, an advisory firm in artificial intelligence, expressed that the mentorship initiative is his contribution to society.
The specialist advised young individuals keen to participate in the three-month mentorship program to express their interest.
PAY ATTENTION: Unlock the best of Legit.ng on Pinterest! Subscribe now and get your daily inspiration!
Source: Legit.ng